How to leverage on AI in your organization
One misconception that exists in the minds of many managers is that AI is a decision maker that provides end-all answers and the functionality will cut costs or reduce the need for costly labor. The reality, however, is that AI remains more of a decision-support mechanism.
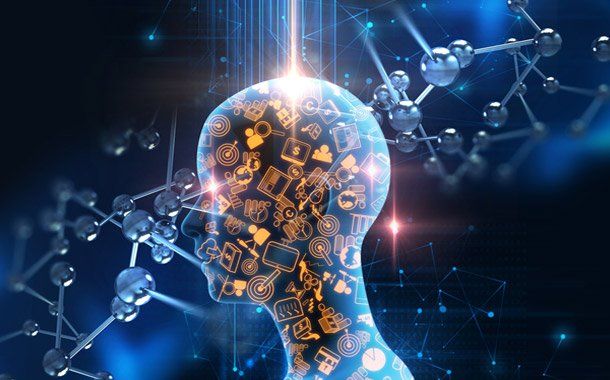
Different forms of AI can improve performance through prediction; automation of routines; and identification of images, keywords, and patterns in voice and text.
However, organizations often struggle with knowing where investments in AI will really pay off. Companies need to:
- Ask whether they really need AI,
- Pick a task to start with, not a project
- Identify what data and complimentary systems it will require,
- Adjust expectations around accuracy accordingly,
- Not rush to deploy it enterprise-wide,
- Ask whether they have the necessary skills to maintain an AI,
- Decide whether the returns will outweigh the costs.
In recent years, AI has become more powerful and its applications to business have increased dramatically. As a result, companies that hadn’t seriously considered using AI are taking a fresh look. The appeal is obvious: different forms of AI can enhance performance through, prediction, automation of routines, identification of images essential to operational activities, or the identification of keywords, phrases and patterns in voice and text for information management.
Where organizations often struggle is in knowing where to invest in an AI project that will really pay off. But if AI hasn’t been a part of your company before, it can be hard to know where the real potential — and risks — lie. While AI may promise valuable gains, those gains come with a price tag, and leaders should feel confident that they’re picking the right project before they commit.
If your company is thinking about adopting AI, you should consider how it might create value, what good first projects might be, and whether you have the right talent on staff for your efforts to succeed in the long run. A first AI project can be daunting, but knowing which factors to focus on will bring the project down to earth — and clarify whether it’s worth the investment at all.
Will AI create value for your company?
Does your company really need AI?
“Why do we think this investment will be worth it?” is one of the first questions you’ll need to answer. That means knowing which source of operational pain (e.g., redundancy in particular tasks or bottlenecks in operational flow) you’re trying to address or where you’re aiming to improve efficiency and innovative competitive advantage (e.g., smart products and smart retailing). AI projects should address processes that significantly impact cost, revenue streams or resource allocations, where the ultimate result can be a noteworthy impact on the bottom line.
Good candidates for AI to provide value include:
- Activities that are very time-consuming and labour-intensive (e.g., reading extensive documentation to categorize its actionable items).
- Workflow activities that require intensive scanning of images.
- Processes that can be augmented with voice analysis (e.g., customer support routing).
- Enhancing predictive accuracy in areas such as customer behaviour or general forecasting, which applies to a multitude of industries such as insurance, finance, marketing, and even agriculture.
Be prepared to accept that AI might not actually be the answer you’re looking for. Even if you find a promising candidate for an AI project, be realistic about where the human element is critical (e.g., validating AI output or red flag verification) and AI may not provide much value. If you plan on making an investment in AI, you want to be sure it will make a difference.
Pick a task, not a project, as your entry point.
AI is generally task oriented. For the first AI experiment at your company, aim to pick a high-value task that is data-driven.
Consider an example from the healthcare sector: identifying patients who may be in a “high probability of falling” category. This is a high-value task because a fall can mean injury to the individual, the need for more complex care, and even legal action. Being able to identify which patients are at risk of falling and adding extra precautionary procedural methods to mitigate the risk of falling can offer real value. It’s a clearly defined task, with a vast amount of data that can be used to train a predictive AI capable of flagging patients as they are admitted for in-patient care.
Know what data and complementary systems you need.
Good data is the lifeblood of a successful AI project. Before committing to a project, you need to formally investigate the types and amount of data required to do it well, whether there are any restrictions on using that data (such as privacy regulations), and whether it’s in a reasonably accessible format. This investigation is not limited to internal data of an organization but data that may exist in external sources. A data engineer can be extraordinarily helpful here. If your data resources are not in order, you may need to focus on data first and pursue AI later.
Once you’ve identified that adequate data is available for AI to process, you’ll need to ensure that it’s possible to integrate an AI’s output into the target task. In other words, can it seamlessly plug into automated operations that depend on its recommendations? If your model is built with Python, will that be compatible with your systems? This is where IT experts play a vital role. It’s a rough awakening when you’ve gone through all the work of creating effective models, only to realize that using the output introduces yet another lengthy and cumbersome project.
Adjust your expectations of accuracy.
AI is a powerful tool, but it’s not magic. The type of AI method you’re deploying, the data you have available, and the task you are looking to focus on can all dictate the rate of accuracy — and the return on investment. Understanding what affects accuracy — and why — can help you set reasonable expectations for what the success of the project looks like. For example, image recognition/computer vision tends to be more reliably accurate than predictive forecasting applications.
Simply put, it’s key to understand the type of AI you are deploying and what the outcomes will be used for in order to estimate the impact on your bottom line.
Don’t rush to deploy enterprise-wide.
Just because an AI works well for one task doesn’t mean it will for others. In other words, consider the task of deploying AI to enhance compliance issues in your organization (e.g. identify sources of non-complaint activities). Models will be effective in identifying red flags according to a particular functional area (booking trades in a fin-tech setting). However, that model will not bplug-and-playplay to other areas to address compliance. AI projects will have to be conducted according to functional procedures and corresponding data that drive them.
Be realistic about whether you have the skills to maintain AI.
Just as processes change, the data that was essential to driving a model one month may be less important a few months later, and this can alter the effectiveness/accuracy of AI. New and more recent data emerges, process drivers change and with that, AI deployments need to be re-optimized. This requires the incorporation of data engineers, data scientists, and IT personnel to provide support for the maintenance of the system to ensure consistently effective AI. This goes to the type of organization. Larger companies already have the infrastructure (e.g. IT and data engineers). The addition of practising data scientists may be enough to maintain internal projects. Of course, the option to engage outside vendors is always a viable play and may be a good way to break into AI deployment and learn.
One notion to keep in mind: if you believe your company has the characteristics that will benefit from AI deployment, it may be worth investing in the personnel to make it an ongoing integral part of your operations.
Will returns outweigh the costs?
One misconception that exists in the minds of many managers is that AI is a decision maker that provides end-all answers and the functionality will cut costs or reduce the need for costly labor. The reality, however, is that AI remains more of a decision-support mechanism. Even with accurate image recognition or effective natural language processing (NLP), an AI initiative will typically enhance stages of a process, and not change the ultimate outcome. For example, NLP can enhance the ability to categorize documents and reduce the need to deploy time and labour-intensive resources to accomplish the task. However, the end result will most likely be a reduction in resources deployed to this task with the excess personnel being deployed to moreknowledge-intensive tasks in the organization. The result is definitely positive, but the ROI is not clear-cut.
AI Projects in Reality
Consider how one Global 500 industrial products company deployed AI to optimize their customer service process regarding customer email interactions.
The need: The company received a large volume of customer service emails. Responding to them involved repetitive processes, which made the task a promising candidate for AI.
The method: The initial project would use natural language processing (NLP) and custom-built classifiers to determine how to route customer emails. This would eliminate the time-consuming and error-prone task of assigning emails to the correct department by staff. It was expected that this would speed up response time by as much as 50% and increase the number of inquiries each customer service agent could handle. In order to move forward, collaboration was established between data scientists, IT personnel and data engineers.
The data: This was a natural entry point as the company had amassed tens of thousands of human-classified emails. This formed a robust training/testing data set that had high accuracy which was a crucial element for this type of project and is often overlooked.
The integration and outcomes: This narrow scope of focus allowed for relatively good accuracy of the classifier; 80% or better. This was selected as the benchmark for classifying inbound emails into one of 10 main categories and then up to 30 subcategories. At this rate, it would be more efficient than human routing and save considerable time and resources. In order to roll the model out into production, data scientists had to implement additional code to integrate the Python model into the existing email-based systems in the organization.
The ultimate result of the project was the demonstration of how AI can impact a time-consuming and labor-intensive business process, which ultimately drove other applications in the organization.
The results of starting AI the “right way.”
The key to a successful implementation of any new strategic technology is due diligence. In the case of AI, which can be highly complex, companies need to know the capabilities of AI methods and consider its deployment to the right processes, ones where it can make a difference. More specifically, due diligence with AI requires collaborative brainstorming among data engineers, data scientists, internal process SMEs and executives. A few days of focused deliberation can enhance the likelihood of successful roll-outs that optimize resources and drive competitive advantage or can avoid the painful purgatory scenario of new technologies remaining in the perpetual state of testing.
If AI is a fit, companies can let the technology do the heavy lifting of routine-based processes or identify seemingly unknown patterns in vast data resources. But in order to get there, you need to find the approach that works for your company. Once you do, you may see new opportunities opening up all around you.
***
Alessio De Filippis, Founder and Chief Executive Officer @ Libentium.
Founder and Partner of Libentium, developing projects mainly focused on Marketing and Sales innovations for different types of organizations (Multinationals, SMEs, startups).
Cross-industry experience: Media, TLC, Oil & Gas, Leisure & Travel, Biotech, ICT.